Applications of Generative AI in Drugs Development and Materials Discovery: IP Considerations
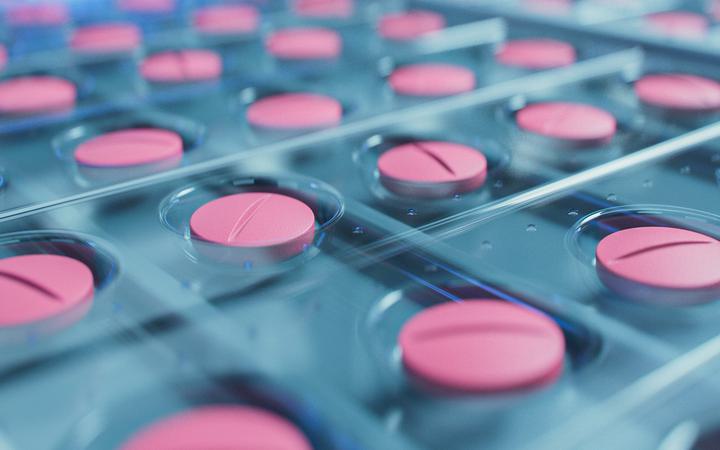
1. Introduction
Artificial Intelligence (AI) is the buzzword of our time. However, the broad concept of AI, encompassing any method that allows machines to imitate human behaviour, has been around since the early 1950s. Already in the late 1990s, machine learning (ML), a subset of AI where computers learn from data, was applied to many problems in academia and industry thanks to the availability of powerful computer hardware. In 2012, the further specialised subset of ML, deep learning (DL), which focuses on complex data modelling using multi-layer neural networks, proved to be a breakthrough technology but it is only with the debut of the transformer architecture that the impressive generative AI (genAI) applications which we are now experiencing came along. While genAI is revolutionising various fields, the advancements in healthcare and chemistry are massive. The ability of genAI to analyse vast amounts of data and generate predictive models has significantly accelerated drug discovery and materials development. This article reviews some genAI applications in these fields and dives into associated intellectual property (IP) issues.
2. Applications of genAI in Drugs and Materials Discovery
AI has transformed drug discovery by enabling rapid identification of potential drug candidates. Traditional drug discovery processes are lengthy and costly, often taking over a decade and requiring billions of dollars to bring a new drug to market. AI can accelerate this process by analysing large datasets of chemical compounds and biological data to predict effective and safe compounds for specific diseases [1].
AI’s impact on the pharma industry does not stop with drug discovery; for example, AI has already been effective in clinical trial design and monitoring, pharmaceutical product development and management, pharmaceutical manufacturing, and quality assurance & control [2]. A high-profile example is the case of Pfizer’s PAXLOVID, a medicament for the treatment of Covid 19, approved by the FDA since 2023. The PAXLOVID development team used real-time predictive models to forecast the virus’ prevalence at a county level, identifying where the next big wave of infections would hit to optimise their selection of clinical trial sites based on where they anticipated recruitment being strongest. In this way, PAXLOVID clinical trials with 46,000 participants at 150 sites in six countries could be launched in just four months.
NVIDIA's contributions to AI have significantly impacted these fields. Their GPUs and AI frameworks enable researchers to process vast datasets and perform simulations much faster than traditional methods, facilitating the development of high-performance genAI applications. Deloitte leveraged massive multimodal datasets to improve preclinical models and target validation, utilising NVIDIA’s NIMs and BioNeMo’s LLM for generating and optimising novel compounds. This approach cut training times dramatically and enhanced researcher productivity by 50%. AI's ability to predict molecular properties accurately can reduce the typical 10-15 year timeline and the high costs associated with drug development.
Exscientia, a prominent AI-driven pharmatech company spun off from Oxford University in 2012, has also made significant strides in this area. By harnessing the power of AI, Exscientia has been able to rapidly discover and develop drug candidates, leading to several promising compounds advancing to clinical trials.
XtalPi is an innovative research platform company powered by artificial intelligence (AI) and robotics. Established in 2015 by three postdoctoral physicists at MIT, and headquartered in China, XtalPi combines quantum physics, AI, cloud computing, and large-scale robotics to provide R&D solutions, services, and products for pharmaceuticals, biotechnology, renewable energy, and advanced materials industries.
In materials science, AI algorithms predict the properties of new materials based on their chemical composition and structure, enabling efficient identification of promising candidates. An example is the work by Szymanski et al. which demonstrates AI's use in automating the synthesis and testing of new materials. AI-driven approaches in materials discovery can lead to breakthroughs in developing more efficient batteries, stronger and lighter materials, and new catalysts for chemical reactions [3].
3. IP considerations for AI related inventions
The rapid integration of AI technologies in drugs and materials discovery brings complex intellectual property (IP) challenges, particularly concerning ownership, the right to use AI technologies and effective protection.
3.1 Right to use and ownership
In AI-driven projects, life sciences and materials companies will typically need to engage in research agreements or partnerships with an “AI provider” company, and multiple IP issues may arise during this process. Clear IP ownership agreements are crucial to avoid disputes and to ensure that all parties benefit fairly from their contributions.
Companies should identify these IP issues as early as possible, ideally before the project starts. Any agreement should define:
- Ownership of preexisting IP, data, AI system and know-how;
- Ownership rights of newly generated IP, data or AI-created inventions;
- Parties’ rights upon termination of the collaboration.
Non-disclosure agreements are also a critical aspect of any joint venture, especially if trade secret protection is contemplated.
The right to use AI technologies involves the permissions granted to various entities to utilise specific AI tools, algorithms, or data sets. This right is often outlined in licensing agreements that specify the terms under which an AI technology can be used. For example, a pharmaceutical company may obtain a license to use a proprietary AI algorithm to accelerate drug discovery processes. The terms of use may include limitations on the scope of use, geographical restrictions, and duration of the license. Licenses can be exclusive or non-exclusive. The right to use AI technologies must be clearly defined to prevent unauthorised use and to protect the Intellectual Property (IP) of the developers.
In collaborative projects between AI companies and other firms, ownership agreements may stipulate that the AI company retains ownership of the algorithm and training data, while the life sciences/materials company owns the resulting drug candidates or materials discovered using the AI system.
As an example, Exscientia typically retains ownership of its AI platform and the algorithms developed. The pharmaceutical partners gain rights to use the AI-discovered compounds and potential co-ownership of any resulting IP. Likewise, XtalPi generally retains ownership of its AI models and computational methods. Partnering pharmaceutical companies often secure exclusive rights to the AI-predicted drug candidates, with agreements outlining IP sharing, development responsibilities, and financial arrangements such as profit sharing and royalties.
3.2 Protection Strategies
Due to the prohibition on patenting abstract ideas, acquiring meaningful patents on artificial intelligence systems is not straightforward. Thus, companies need to carefully consider alternative protection options and align their strategy with their business objectives.
For AI-related inventions there are typically three primary blocks for which protection should be considered: core AI algorithm; training methods; and AI output.
3.2.1 Algorithms and Training Methods
For AI algorithm and training methods, the question arises whether they should be placed in the public domain, be kept proprietary as a trade secret or be patent protected.
Open-source algorithms are made available to the public, allowing anyone to use, modify, and distribute the code, fostering innovation and collaboration. However, it can be challenging to monetise open-source algorithms directly, and there is a risk that competitors could use the technology without providing compensation. Proprietary algorithms are kept confidential or protected through IP laws.
At the European Patent Office (EPO), the patentability of AI algorithms and training methods hinges on their technical character and contribution to solving a technical problem. The EPO introduced updated guidelines in 2018 which contained guidance on the patentability of AI and machine learning, a new version of which was published on April 1, 2024. These EPO Guidelines (G-II, 3.3.1) define AI and machine learning as being based on:
“…computational models and algorithms for classification, clustering, regression and dimensionality reduction, such as neural networks, genetic algorithms, support vector machines, k-means, kernel regression and discriminant analysis.”
Such computational models and algorithms are per se of an abstract mathematical nature and, as such, excluded from patentability, unless they solve a technical problem.
Examples of technical contributions include improvements in data processing efficiency, novel machine learning techniques, or enhancements in computer hardware performance. EPO Boards of Appeal decisions T 874/19 and T 1635/19 provide two examples wherein a link to a technical effect could not be found and patents were consequently refused.
When considering the patentability of training methods for AI algorithms in Europe, several additional important factors must be taken into account. These include the clarity of the claims, the plausibility of the described methods, and the sufficiency of disclosure.
Clear claims help delineate the novel aspects of the training method and how they differ from existing techniques. The specific steps and processes used to train the AI model should be unambiguously defined. EPO Boards of Appeal decision T 1882/17 shows a case wherein it was impossible to determine what terms in the claims meant.
Sufficiency of disclosure requires that the patent application provides enough information for a person skilled in the art to replicate the invention. For training methods, this involves a detailed description of the data used, preprocessing steps, model architecture, training algorithms, and optimisation techniques. The disclosure should be comprehensive enough to allow others to understand and implement the training method without undue experimentation (see, for example, T 509/18, T 161/18 and T 1191/18).
The described methods must also be credible and plausible, supported by enough detail and evidence to demonstrate they are more than speculative or hypothetical ideas (T 2147/16).
Therefore, if patent protection is envisaged, careful thought must be given to the following points:
- The application must be drafted to ensure that the AI is tied with technical features.
- The mathematical method, training process must be adequately described.
- Suitable training data and training algorithms should be described, and sample training data should be provided if possible.
When seeking patent protection, it should also be considered that each jurisdiction has their differences, particularly concerning what constitutes a patentable AI invention, making it crucial to tailor patent applications to meet the specific requirements of each region.
China is generally considered the most favourable jurisdiction for patenting AI-related inventions due to its broader interpretation of what constitutes a patentable technical solution. The United States (USPTO) presents more challenges due to its strict interpretation of patentable subject matter, particularly when dealing with abstract ideas and algorithms. The USPTO recently issued guidance on the patentability of AI. The new guidance seeks to provide some clarity and includes some practical examples relating to AI and its use in precision medicine. With a well-crafted application, it is still possible to secure patents for AI-related inventions in the US, especially when the AI is applied in a novel and non-obvious way to solve technical problems.
Due to the existing challenges in relation to patents on artificial intelligence systems, companies are increasingly turning to trade secret protection to protect their AI-related intellectual property.
Unlike patents, trade secret protection can be obtained without any application or registration. It arises automatically if the trade secret owner takes appropriate steps to ensure the information is kept secret for as long as the secrecy constitutes a commercial advantage. Examples like Google’s search algorithm demonstrate how trade secrets can be a powerful form of IP protection. However, in many respects, trade secret law provides far weaker protection than patent law. While trade secret law does not forbid the discovery of the trade secret by fair and honest means, such as independent creation or reverse engineering, with patents, any use of the invention can be limited for a certain length of time. Trade secrets are also susceptible to being passed on to competitors by theft or by breach of a confidential relationship in a manner not easily discoverable.
3.2.2 AI Output
In theory, AI output such as a new compound or a new crystal, is subject to known and existing patenting requirements. In practice, some unique challenges arise around inventiveness, inventorship, and the acceptance of in silico data.
For example, the question arises whether drug structures or medical uses suggested by AI can be considered “inventive”. The mere fact that AI was used to find a solution does not guarantee that the solution would not have been obvious to a skilled person or skilled team. It is, therefore, still important for applicants to conduct the same patentability analysis as for non-AI-based applications before filing.
As to inventorship, the Legal Board of Appeal of the EPO has issued its decision in case J 8/20, which confirmed that under the European Patent Convention (EPC), an inventor designated in a patent application must be a human being. The decision concerned the rejection of two European patent applications in which an artificial intelligence system called DABUS was designated as inventor. Similar approaches have been confirmed in the US, UK and Germany.
This often leads to the developers or users of the AI being named as inventors, provided they can demonstrate a significant contribution to the inventive process. As AI technology evolves, legal systems will need to adapt to address these challenges more explicitly.
In silico data refers to data generated by computer simulations or models, rather than through physical experimentation. This type of data is increasingly common in fields like drug discovery and materials science, where AI can model complex interactions and predict outcomes with high accuracy. Patent offices traditionally require empirical data to support the claims made in a patent application. As in silico data becomes more reliable and widely used, there is a growing push for its acceptance as valid evidence. Applicants must provide robust validation of their in silico models, demonstrating that the data accurately represents real-world phenomena.
Due to these uncertainties, it is advisable that applicants keep a conservative approach by including as much data as possible in initial filings, preferably including in vitro and in vivo data wherever possible. Applicants should also ensure that any description of required computational methods contains enough detail to enable a skilled person to reproduce the method, and any training data should be described as thoroughly as possible. However, before disclosing any aspect of a proprietary AI-based method, applicants should carefully consider the risks entailed.
4. EU AI Act
The EU AI Act , the first comprehensive regulation on AI, has significant implications for intellectual property and patents, although it does not directly address patent law. For example, the AI Act mandates the disclosure of copyrighted works used in training and may set indications for defining what will constitute patentable subject matter at the EPO. Although the European Patent Office (EPO) is not bound by EU regulations, it is expected to adopt a similar approach, potentially excluding some AI technologies from patentability.
As AI technology advances, so do the legal frameworks governing its use in Europe and elsewhere. Companies must stay informed about changes in IP laws and adjust their strategies accordingly to maintain compliance while protecting their interests.
5. Conclusion
GenAI is revolutionising Drugs and Materials Discovery with a massive impact expected by 2030. Companies active in these fields should therefore invest time to understand who the players are in this area, and what each of them brings to the table. Clear ownership/license agreements are crucial to avoid disputes and to ensure that all parties benefit fairly from their contributions. Specific IP considerations and challenges exist for AI-related inventions, and companies should make sure to consider them for maximising protection and return. By understanding and addressing these IP considerations, organisations can harness the full potential of generative AI while safeguarding their technological advancements and competitive edge.
As the legal landscape evolves, staying informed about IP considerations and regulatory changes will be crucial for maximising protection and return on AI-related inventions.
And for anyone feeling threatened by the AI revolution it should always be kept in mind that: “together the human and AI can outperform any human, but they can also outperform any algorithm.” [1].
References
[1] Nature Vol 549, P. 445-447, 2017
[2] Drug Discovery Today Volume 26, Number 1 January 2021, p 80-93
[3] Nature, Vol 624, P 86-87, 2023